Tracktest English Test validity study
The measurement of expert agreement with the computer-aided testing result
Project details
Institution: theBridge Language School, Bratislava, Slovakia
Participants: 15 teachers and 135 adult students of English as a foreign language (EFL)
Dates: June-August 2018
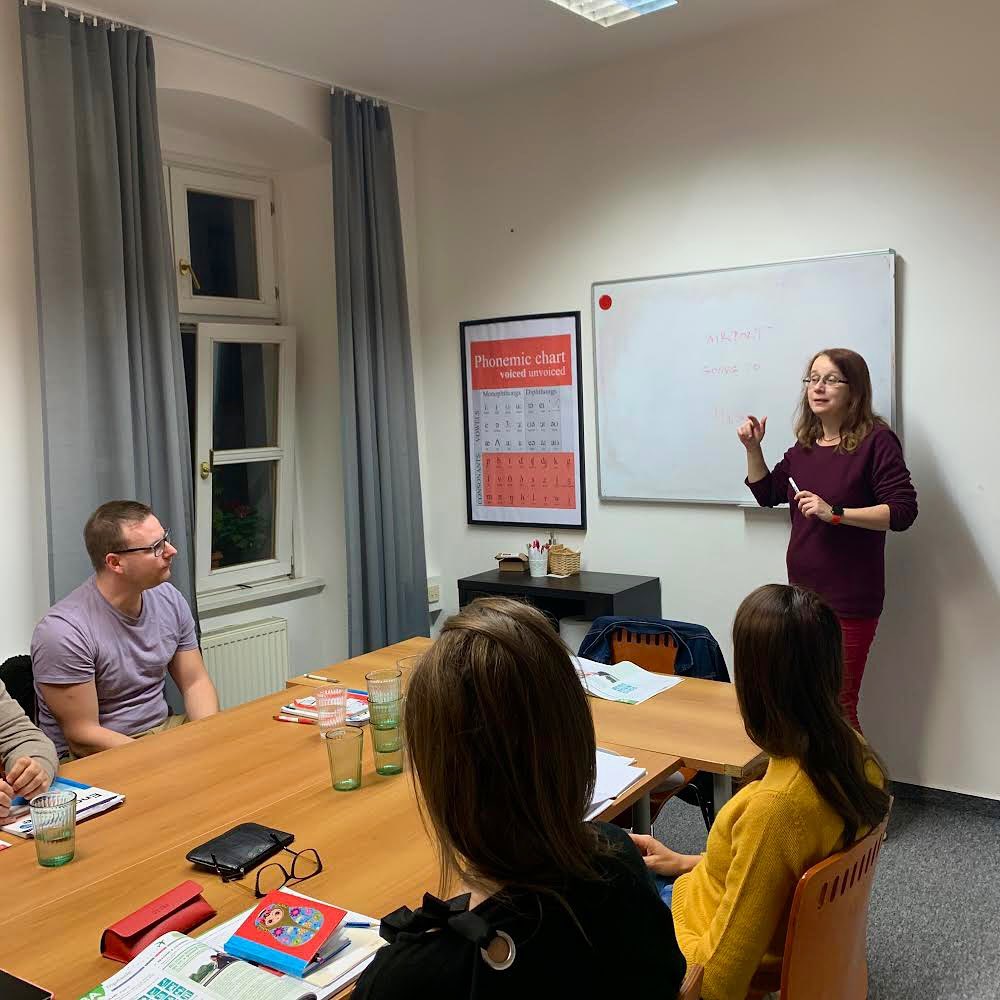
Construct validity is an important measurement of the quality of a language proficiency test’s usefulness (together with reliability, authenticity, interactivity, impact, and practicality, see Bachman and Palmer 1998). It is expected that the automatic ratings of the computer-based testing system are in accordance with the assessment provided by human subject-matter experts. It can be demonstrated in the classification of test-takers into the appropriate CEFR level (A1 to C2; see Osborne 2014).
In our study, a sample of adult students (Ntotal = 135) completed the TrackTest English Proficiency Core Test (Use of English, Reading, Listening). As a method of the TrackTest validation, we used the external assessment of student’s performance by the experienced raters. Each student was assessed by one out of 15 experienced teachers of English as a foreign language (EFL).
The students were distributed among raters unevenly, the number of students assessed by each rater varied from 3 to 23 with a median number of test takers assessed by one rater = 7.
Comparison of Tracktest and language school experts’ assessments with weighted Cohen’s kappa used as a measure of the criterion validity demonstrated substantial to an almost perfect agreement (Kappa(weights: squared) = .826 (p < .001).
Both the TrackTest assessment algorithm, as well as the expert raters, classified the students into one of 6 CEFR levels. Besides of percentage efficiency at the specific CEFR level, the Tracktest algorithm also estimates the overall proficiency score (further mentioned as the Tracktest Score, TTS) independent of the CEFR level. The same percentage of correct answers in a test at each CEFR level is expected to correspond to the rising TrackTest Score. This scale will be used to compare the rater’s assessment of the CEFR level attained by the students and their TrackTest Score.
The successful attainment of the given CEFR level by the Tracktest system is indicated by the reaching of the cut-off value defined as 65 % of correct answers. As expected, not everyone from the sample passed the TrackTest Core test. Because the expert raters were not informed about the test result and were instructed to assess the level really attained by the student, for the sake of analysis we consider only those students passing the TrackTest on the corresponding level. The sample size of students passing the test was Nred = 108.
Considering that both TrackTest System and the experts provide the classification of the student into one of six levels according to the CEFR, we can use the estimate of an agreement between these two ratings (i. e. the interrater reliability index) as a measure of criterion validity (Cohen 1968). This approach is widely used in the validation of computer-based L2 testing (for example Risdiani 2016) and in other contexts, predominantly in medical sciences (del Mar Seguí, Cabrero-García, Crespo, Verdú, & Ronda 2015).
On a descriptive level, we can visualize the agreement between Tracktest software and the human rater as a contingency table (Tab. 1).
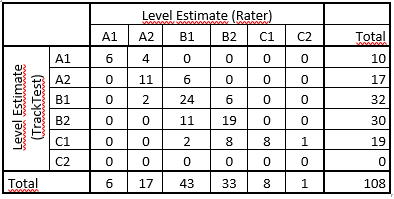
From Tab 1 we may conclude that there is quite a visible agreement between the testing software and human raters. In most cases (68 out of 108), the students obtained the same rating, i. e. they were assessed to achieve the same CEFR proficiency level. As a first proxy of the consistency between the classification based on the TrackTest System and the human rater, the Spearman rank correlation of the TrackTest Score and the rater’s assessment of the achieved level was calculated. The Spearman rho = .723 (p < 2.2e-16) indicates the relatively high level of consistency of these two kinds of assessment. This result is demonstrated by the boxplot (Fig. 1).
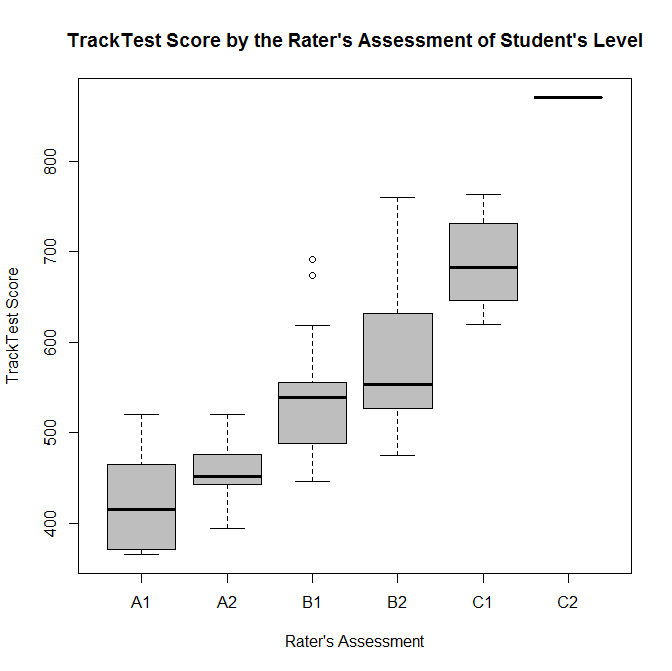
However, for a more formal assessing the agreement between the software and the expert, we need to consider also the cases of disagreement. Cohen’s kappa is the most common method used for this task. It is an index of interrater reliability which considers the amount of agreement above the random level (Cohen 1960; 1968). To estimate Cohen’s kappa and to provide a significance test of a null hypothesis concerning kappa, we used the relevant functions of the package irr (Gamer, Lemon, & Singh 2019) available in the statistical environment R. Regarding the appropriate statistical power, we calculated the expected sample size using the marginal probability estimates from the crosstab (Tab. 1). For the null hypothesis that kappa < .4 (with an alternative hypothesis that kappa > .6), significance level alpha = .05 and statistical power = .8, the minimal sample size needed is Nmin = 84. Our sample of N = 108 meets this requirement.
Although in most cases of disagreement, the ratings differed only by one level, there were two cases where the difference between the two ratings reached two levels. It was the case where the TrackTest System classifies the student as achieving the C1 level whereas the expert rates the same student as achieving B1 only. Because we want to penalize such large disagreements, the weighted kappa was used (Cohen 1968). We decided to use both squared and equal weights to prevent the overestimation of the agreement. When we use the squared weights, the disagreements are weighted according to their squared distance from the perfect agreement. Otherwise, all disagreement is weighted equally. Under the quadratic weighting, Kappa(weights: squared) = .826 (p < .001). However, if we weigh the disagreement equally, Kappa(weights: equal) = .682 (p < .001).
These results show that weighted Cohen’s kappa used as a measure of the criterion validity for the Tracktest Core test against human experts demonstrates substantial to the almost perfect agreement (Landis & Koch 1977).
References:
- Bachman, L.F. & Palmer, A.S. (1996). Language testing in Practice. Oxford: Oxford University Press
- Cohen, J. (1960). A coefficient of agreement for nominal scales. Educational and Psychological Measurement, 20, 37-46.
- Cohen, J. (1968). Weighted kappa: Nominal scale agreement with provision for scaled disagreement or partial credit. Psychological Bulletin, 70, 213-220.
- del Mar Seguí, M., Cabrero-García, J., Crespo, A., Verdú, J., & Ronda, E. (2015). A reliable and valid questionnaire was developed to measure computer vision syndrome at the workplace. Journal of Clinical Epidemiology, 68(6), 662-673.
- Gamer, M., Lemon, J., & Singh, I. F. P. (2019). irr: Various Coefficients of Interrater Reliability and Agreement. R package version 0.84.1. Retrieved from https://CRAN.R-project.org/package=irr
- Landis, J. R., & Koch, G. G. (1977). The measurement of observer agreement for categorical data. Biometrics, 33(1), 159-174.
- Osborne, J. (2014). Multiple assessment of oral proficiency: Evidence from a collaborative platform. In P. Leclercq & A. Edmonds (Eds.). Measuring L2 proficiency. Perspectives from SLA (pp. 54–70). Bristol: Multilingual Matters.
- Risdiani, R. (2016). Placement testing in computer-assisted language testing: Validating elicited imitation as a measure of language proficiency. Nijmegen: Radboud University Nijmegen.